"Inferences from the State Meet"
https://dop3o1hd82eb7.cloudfront.net/user_files/11171/131296/state_analysis2x.pdf
I track about 160 athletes (all boys) during a cross country season. After a few weeks, I can compute a time adjustment for each event that normalizes the data. Once I have that normalization factor, I can predict a time for each boy on a given course if they run a course that is in the database. Carrollton is in the database after the Carrollton Orthopedic Invitational (COI). Knowing the adjustment factor for that event allows me to predict times at state for each boy regardless of whether that boy actually ran the COI. Actual times at state will be faster than predicted for some boys and slower than predicted for others.
This study began as an attempt to determine if running a fast course, such as ASICS, helped or hindered performances at the state meet. I could not see where it had any impact on performances at state as the deviation from predicted was not statistically significant between the group that ran ASICS and the group that did not. I did see noticeable team-to-team variations in performance vs. predicted.
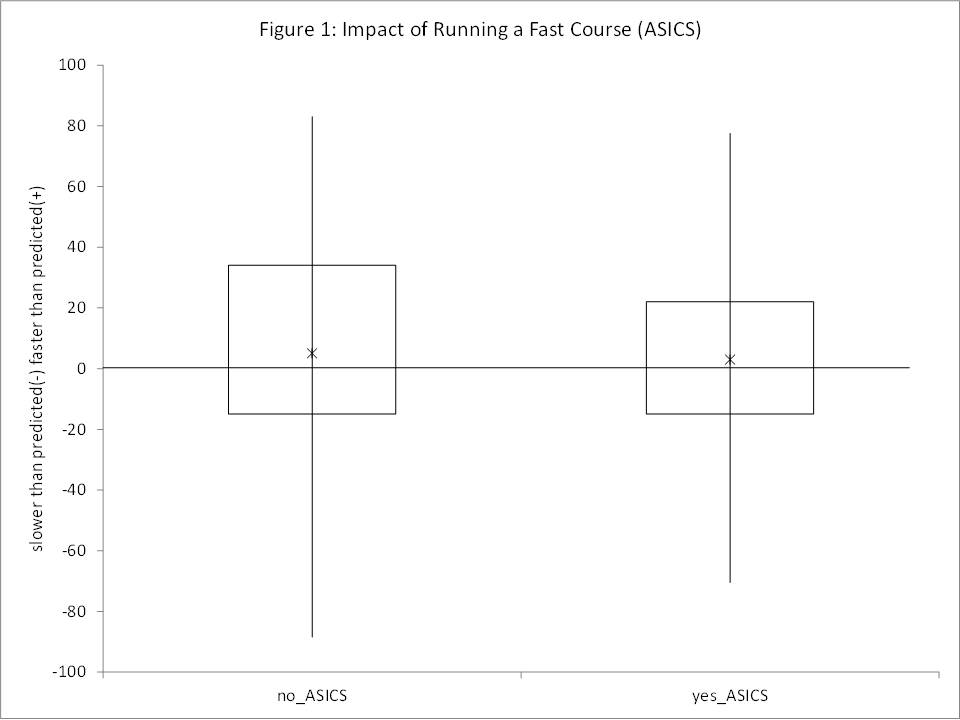
The lack of impact of ASICS is depicted in Figure 1. Some of you may not be familiar with boxplots. A boxplot is a method to depict a distribution showing the median (the asterisk), the 5th percentile (bottom of whisker), 25th percentile (bottom of box), 75th percentile (top of box), and 95th percentile (top of whisker). Note that in Figure 1, the “no_ASICS” box is almost identical to the “yes_ASICS” box, indicating that whether or not a team that ran that particular meet was not a good predictor of performance at state.
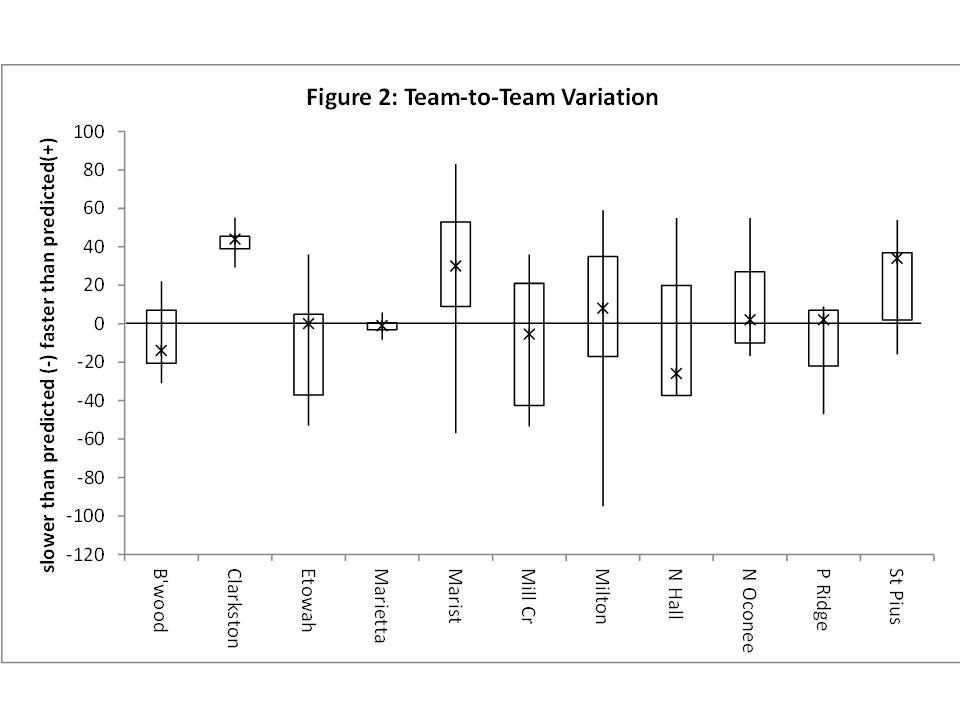
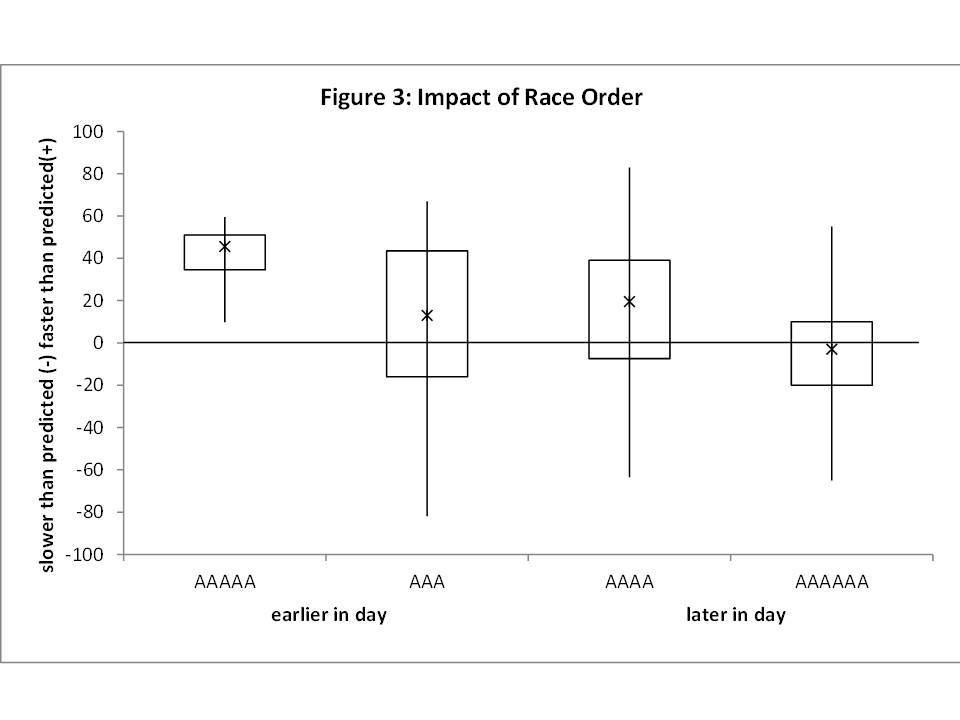
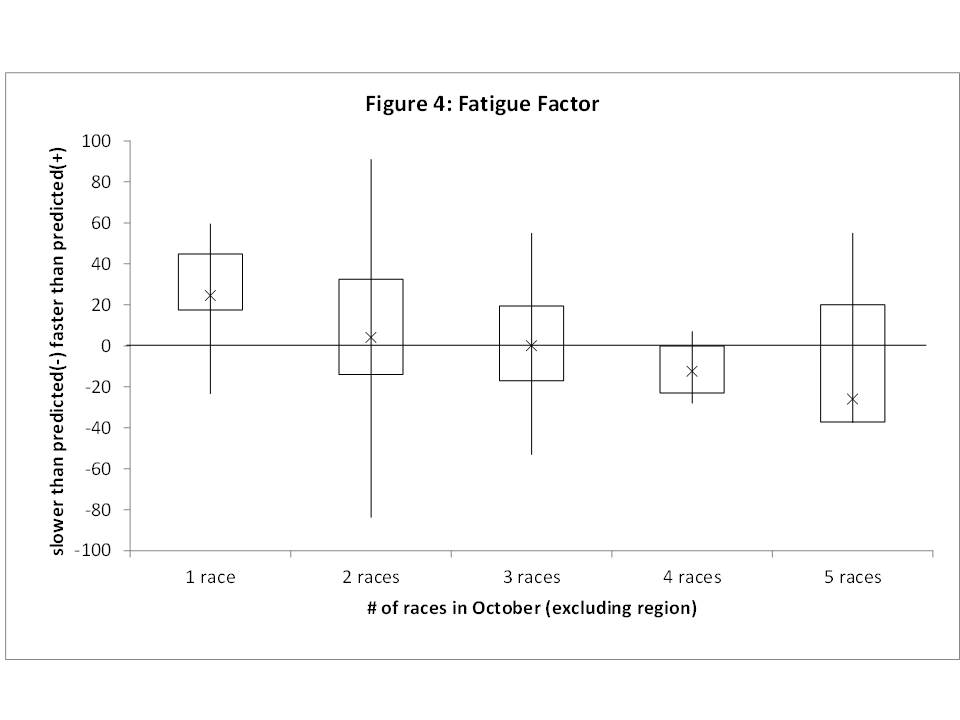
Putting it all together, we would expect a team running in race #1that had only had one October meet to run 80 seconds faster at state than would another team with equivalent ability that was running in race #9 and had run five meets in October. Of course a coach does not choose the order of races at state, but he can choose the number of races to run in October. I can’t say for sure that running one October race is preferable to two, but I would not advise running more than two October races if winning state is a team goal.